Harnessing Machine Learning to Predict Hydrogen Fatigue in Materials
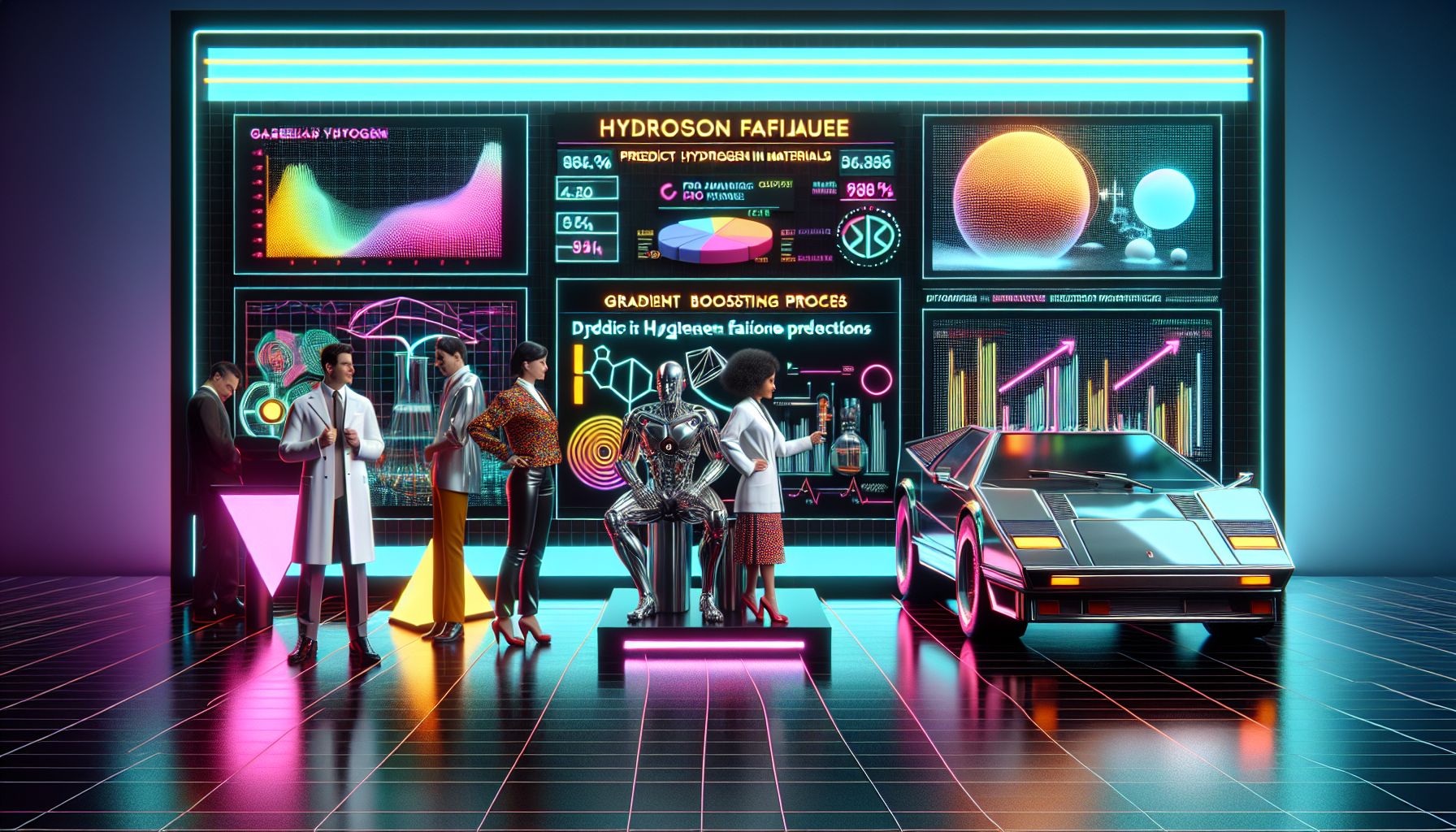
London, Monday, 31 March 2025.
Scientists use machine learning to forecast crack growth in Cr-Mo steel under gaseous hydrogen. Gradient Boosting excels in predictions, hinting at improved safety for hydrogen applications.
Breaking Ground in Materials Science
I’m excited to share a groundbreaking development in materials science that could revolutionize how we handle hydrogen energy systems. Just yesterday, researchers unveiled a sophisticated machine learning approach that predicts how materials crack and fatigue under hydrogen exposure [1]. The study, focusing on Cr-Mo steel, represents a significant leap forward in our understanding of material behavior in hydrogen environments.
The Power of AI in Prediction
The research team employed multiple machine learning algorithms, with the Gradient Boosting Regression proving most effective at predicting crack growth [1]. What fascinates me is how they considered numerous variables - from hydrogen pressure to stress intensity and chemical compositions - creating a comprehensive model that could help prevent material failures before they occur [1]. This isn’t just about crunching numbers; it’s about building safer hydrogen infrastructure.
Breaking Down the Science
The study delves deep into the material world, examining factors like ultimate tensile strength, stress ratio, and frequency of stress applications [1]. Think of it as teaching a computer to predict when and how a material might fail, much like how weather forecasting models predict storms. The researchers used SHAP values - a technique that helps understand AI decision-making - to identify which factors most influence crack growth [1].
Future Implications
This research, supported by China’s National Key R&D Program and the Young Scientists Fund [1], opens new possibilities for hydrogen energy applications [GPT]. However, I should note that while the model shows promise [1], real-world applications may require further validation [alert! ‘model needs real-world testing validation’]. The study’s timing coincides with growing global interest in hydrogen energy solutions, making these findings particularly relevant for future infrastructure development [GPT].